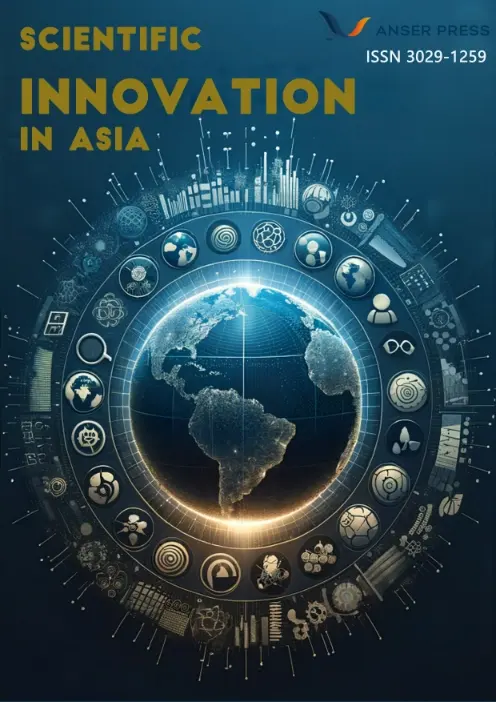
Volume 3, 2025 - Issue 1
Artificial Intelligence: Impact on the Development of Electrical Materials
Abstract
Electrical materials play a pivotal role in electrical engineering, yet conventional research methodologies relying on experimental approaches and theoretical calculations face challenges of low efficiency and high costs. The rapid advancement of artificial intelligence (AI) technology has introduced novel tools for electrical material research, significantly enhancing the efficiency of material design, performance prediction, and experimental optimization. This paper systematically reviews recent progress in AI applications across semiconductor, alloy, energy storage, and metallic materials. In semiconductor research, machine learning has improved analytical precision through optimization of Hubbard U parameters and prediction of perovskite defect transition levels. For alloy materials, AI combined with active learning strategies has accelerated the discovery and performance optimization of high-entropy alloys.Our analysis reveals that AI technology substantially reduces material development cycles and experimental costs through multi-source data integration, quantitative model construction, and multi-objective optimization strategies. Looking forward, with advancements in data science and computational capabilities, AI is poised to play an increasingly critical role in multi-scale material design, performance prediction, and novel material development for electrical engineering, thereby driving technological innovations in power and electronic systems.
Share and Cite
Article Metrics
References
- Liu Hengyu. Research on Properties of III-V Semiconductor Materials Based on Machine Learning [D], 2023.
- Wu Xiaoyu. Theoretical Prediction of Perovskite Defect States Based on Machine Learning [D], 2023.
- Hosseini ,et al. Machine Learning-enabled Prediction and Optimization of Hardness for Nb-Ti-V-Zr Refractory High Entropy Alloy [J]. Materials Today Communications, 2024, 40.
- Rao z, et al. Machine Learning-enabled High-entropy Alloy Discovery [Z]. arXiv, 2022.
- Zhao C, et al. Machine-learning for Designing Nanoarchitectured Materials by Dealloying [J]. Communications Materials, 2022, 3(1).
- Xu Jing, et al. Design of New Battery Materials Based on Big Data [J] Energy Storage Science and Technology [J]. 2024, 13(09): 2920-32.
- Deng B, et al. CHGNet as a Pretrained Universal Neural Network Potential for Charge-informed Atomistic Modelling [J]. Nature Machine Intelligence, 2023, 5(9): 1031-41.
- Lu C et al. Machine Learning: An Advanced Platform for Materials Development and State Prediction in Lithium-Ion Batteries [J]. Adv Mater, 2022, 34(25): 17.
- Cai ,et al. Prediction of Contact Resistance of Electrical Contact Wear Using Different Machine Learning Algorithms [J]. Friction, 2024, 12(6): 1250-71.
- Zhi shan,et al. Effect of Carbon Content on Hydrogen Resistance of Steel Materials Based on First-principles and Machine Learning [J] Journal of Metal Functional Materials [J]. 2024, 31(01): 44-50.