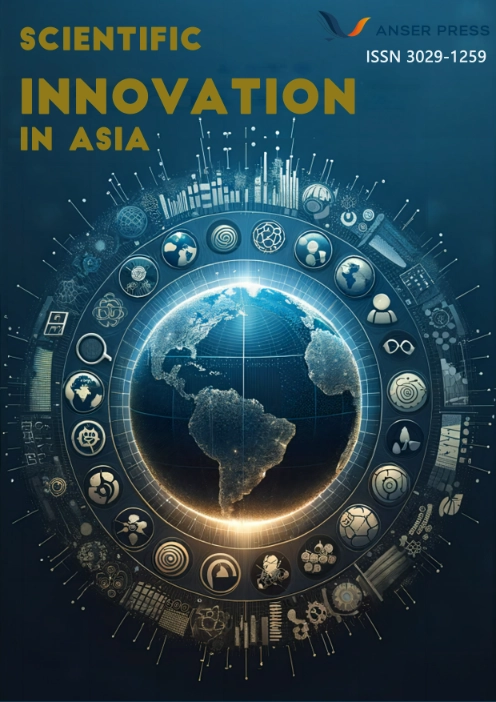
Volume 2, 2024 - Issue 1
Current Research Status of Student Performance Analysis in the Context of Big Data in Higher Education
Abstract
Big data in education has become a driving force for educational transformation and innovation, emerging as a prominent area of focus in educational technology research. In the context of big data in higher education, student performance analysis is a key issue that has garnered significant attention from researchers and institutions alike. This review provides a systematic overview of student performance analysis from the perspective of educational data mining. The process of student performance analysis is categorized into three steps: the acquisition and preprocessing of educational big data, methods for analyzing student performance, and the visualization and application of data analysis results. Various methods for student performance analysis and their applications are introduced in detail, summarizing existing research and providing insights into future research directions.
Share and Cite
Article Metrics
References
- Baker, R. S., & Inventado, P. S. (2014). Educational data mining and learning analytics. Learning analytics, 61-75.
- Romero, C., & Ventura, S. (2010). Educational data mining: A review of the state-of-the-art. IEEE Transactions on Systems, Man, and Cybernetics, Part C (Applications and Reviews), 40(6), 601-618.
- Siemens, G., & Long, P. (2011). Penetrating the fog: Analytics in learning and education. EDUCAUSE review, 46(5), 30.
- Dutt, A., Ismail, M. A., & Herawan, T. (2017). A systematic review on educational data mining. IEEE Access, 5, 15991-16005.
- Aluko, F. R. (2009). The impact of engagement with course resources on academic performance: A study of higher education distance learners. Studies in Higher Education, 34(6), 735-747.
- Wang, Y., & Wu, Y. (2019). Early prediction of student learning outcomes with deep learning and data mining techniques. Journal of Educational Data Mining, 11(1), 28-49.
- Lu, O. H., Huang, A. Y., Huang, J. C., Lin, A. J., Ogata, H., & Yang, S. J. (2018). Applying learning analytics for the early prediction of students' academic performance in blended learning. Journal of Educational Technology & Society, 21(2), 220-232.
- Yu, Z., & Li, M. (2020). Big data analytics and its applications in education: Concepts, technologies, and trends. Journal of Data Science, 18(1), 1-14.
- Tempelaar, D. T., Rienties, B., Giesbers, B., & Gijselaers, W. H. (2015). The pivotal role of motivation and emotion in learning analytics: Predicting academic success and dropout. British Journal of Educational Technology, 46(6), 1236-1250.
- Bhardwaj, A., Tiwari, A., & Kalra, R. (2019). Predicting students' performance using machine learning techniques. Journal of Educational Technology & Society, 22(3), 24-36.
- Pardos, Z. A., & Dadu, A. (2017). Early detection of students at risk of failing: A machine learning approach. International Educational Data Mining Society.
- Prinsloo, P., & Slade, S. (2016). Student vulnerability, agency, and learning analytics: An exploration. Journal of Learning Analytics, 3(1), 159-182.
- Reddy, V. R., & Srinivasan, K. (2018). Predictive analytics in education: Machine learning techniques for student performance prediction. Journal of Educational Data Mining, 10(3), 45-59.
- Zhang, T., Liu, Q., & Zhang, X. (2020). Educational data mining methods for discovering learning patterns. Journal of Information Science, 46(4), 549-565.
- Luan, J., & Zang, H. (2021). The impact of early engagement on the academic success of undergraduate students. Educational Research Review, 30, 100385.
- Wang, Z., & Yu, J. (2019). Exploring deep learning for predicting student success in higher education. IEEE Access, 7, 176929-176940.
- Nguyen, A., & Gardner, L. (2020). Predicting student performance in higher education: A review of decision tree classification methods. Journal of Educational Data Mining, 12(3), 1-20.
- Ali, L., Hatala, M., Gašević, D., & Jovanović, J. (2012). A qualitative evaluation of evolution of a learning analytics tool. Computers & Education, 58(1), 236-249.
- Liaw, S. S., & Huang, H. M. (2013). Exploring learners' acceptance toward mobile learning in a social media context: A structural equation modeling approach. International Review of Research in Open and Distributed Learning, 14(2), 79-100.
- Horváth, C., & Gergely, M. (2020). Learning analytics and early identification of students at risk of failing in higher education. Journal of Educational Data Science, 8(2), 143-156.
- Kumar, V., & Sinha, S. (2018). Student performance prediction in higher education using machine learning algorithms. Proceedings of the International Conference on Data Science and Engineering, 98-107.
- Liu, X., & Huang, X. (2021). Analyzing academic performance of students using big data technologies: A review. Computers in Human Behavior, 115, 106606.
- Xia, T., & Lee, W. (2019). Data mining approaches for predicting student performance: A comprehensive review. Educational Data Mining, 11(2), 44-59.
- Chen, Y., & Zhang, Q. (2020). Leveraging big data and machine learning techniques for educational performance analysis. Journal of Educational Technology, 15(4), 223-237.
- Zhou, L., & Wu, C. (2017). A survey on educational data mining and learning analytics. International Journal of Information and Education Technology, 7(5), 367-371.
- Mendez, J., & Garcia, J. (2021). Predicting student dropout in higher education using ensemble methods. Educational Technology Research and Development, 69(2), 291-308.
- Feng, C., & Zhang, H. (2018). Application of machine learning algorithms in predicting student academic performance: A case study. International Journal of Learning Analytics and Artificial Intelligence, 1(2), 15-27.